DOWNLOAD EBOOK
Exploring the Growing Convergence Between Blockchain and AI
And how it supports ethical AI initiatives for businesses
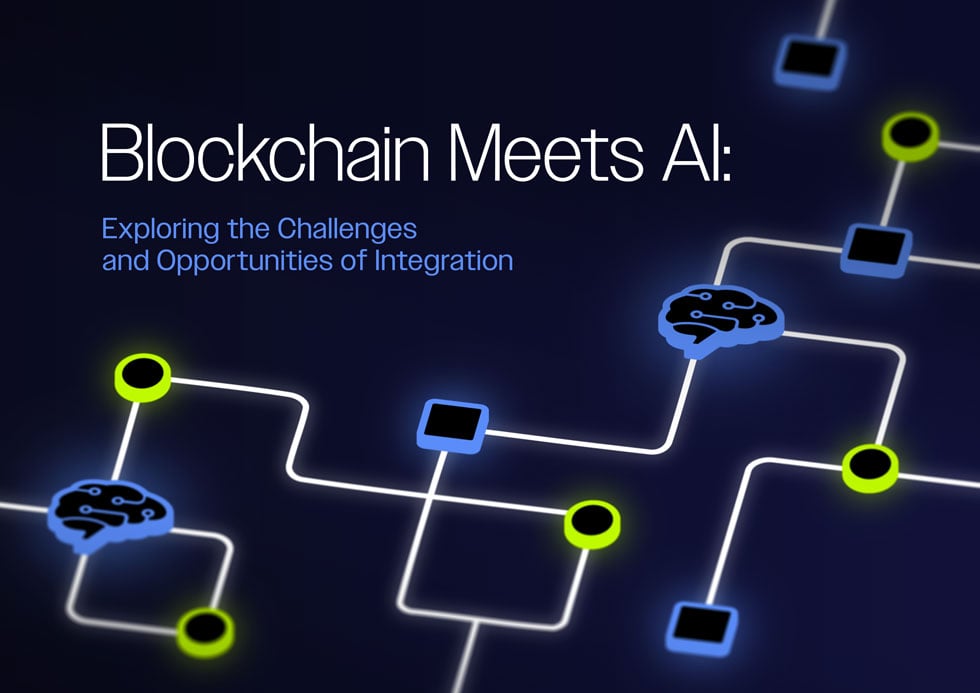
When it comes to modern AI systems and AI risks – and the biggest barriers towards realizing a more responsible approach to AI – three key truths emerge:
- Generative AI is the leading application driving most AI use cases today and in the near future.
- GenAI systems process large data inputs without providing insight into their decision-making process.
- AI systems' data and GPU usage is unsustainable for organizations as AI margins tighten.
Download the eBook for a detailed look into how blockchain technology is increasingly being used to augment AI systems.

Download Now
How to Responsibly Harness AI’s Benefits.
When it comes to modern AI systems and AI risks – and the biggest barriers towards realizing a more responsible approach to AI – three key truths emerge:
- Generative AI is by far the most current popular application of AI, and drives the majority of current and near-term AI use cases.
- GenAI systems today are a black box when it comes to data. This non-deterministic approach relies on raking in a vast amount of inputs, synthesizing them and spitting them out on the other side with no context as to why or how it reached a given conclusion.
- AI systems are consuming a massive and ever-growing amount of data and GPU. Training and re-parameterizing AI systems under the current system is not going to be sustainable for most organizations over the long run, as AI margins inevitably tighten.
For all of its immense promise, AI – especially generative AI – isn’t going to be a feasible option for most businesses until they can more reliably audit and modify AI systems. For a more detailed overview of what the ideal risk management model for AI looks like, it’s worth looking at the NIST’s comprehensive standards framework, which has emerged as a guiding north for the entire industry.
Realizing these standards ultimately comes down to achieving greater transparency into AI training data; today, that environment is essentially a black box. When an AI hallucination occurs, it’s prohibitively difficult if not impossible to verify why and where it happened – which makes addressing the problem a non-starter.
Blockchain technology offers the most cost-effective and tamper-proof approach to realizing this critical level of visibility. When AI is augmented with blockchain, it’s possible to reconcile and track which data caused which outcomes, when and why. It also unlocks version control: when a given AI system falters, there’s currently no reliable method to “restore” a previous, working iteration. Think of this as akin to Google Docs’ beloved “restore previous version” feature, just on a much grander scale.
While the economics of such an approach are challenging in a traditional public blockchain environment, the advent of hybrid blockchains eradicates that concern. By hashing key data and storing it on-chain, organizations retain a tamper-proof methodology, while maintaining sensitive and/or extraneous data in more cost-effective private environments.